Director Message
Welcome to the newest Mitrais Newsletter for 2025. We have a selection of informative articles that we hope that you find interesting.
Firstly, we invite you to discover the future of software development and gain a competitive edge with an insightful article outlining the importance of endpoint security. Remote work, while offering flexibility and convenience, significantly expands the attack surface, making devices like laptops, tablets, and smartphones prime targets for cybercriminals. This article highlights endpoint security strategies for protecting sensitive data, preventing unauthorised access, and maintaining business continuity. Whether you’re a tech leader or a curious enthusiast, this article is your roadmap to staying ahead and embracing the opportunities of tomorrow.
AI is certainly a hot topic everywhere, and Mitrais is no different. Explore how cutting-edge AI technology is transforming Mitrais’ employee engagement and efficiency through this compelling white paper. Discover the innovative use of Large Language Models (LLMs) in enhancing our unique Competency System, leveraging embedded Retriever-Augmented Generation (RAG) and function calling to provide accurate, personalised responses to employee queries. Learn about the technical implementation, challenges, and operational benefits, including improved satisfaction, efficiency, and scalability. This document not only offers valuable insights into integrating AI into HR processes but also sheds light on the future of AI-driven support systems, making it a must-read for forward-thinking organisations.
Our clients are a key asset, and Mitrais has been a trusted and innovative software development partner for Integrated Research (ASX:IRI), a leader in system communication, payment, and infrastructure monitoring. Through our collaboration, Integrated Research significantly boosted development speed while maintaining high-quality standards, thanks to Mitrais’ focus on measurable outcomes and tailored talent solutions. Watch a video interview where leaders from both organisations discuss how Mitrais meets Integrated Research’s unique software development needs.
This month’s final article highlights the inspiring journey of I Putu Arya Wijaya Kusuma, Compliance and Operations Lead at Mitrais. With a background in electrical engineering and multiple cybersecurity certifications, Arya has excelled in IT operations, network monitoring, and ISO 27001 compliance. He values Mitrais’ supportive and collaborative culture, which has enabled his growth and achievements, including overcoming challenges in ISO certification implementation. Arya’s story exemplifies ambition, continuous learning, and teamwork, serving as a testament to how dedication and a nurturing environment can drive success in the IT and cybersecurity fields.
As always, we hope you enjoy this quarter’s newsletter, and we wish you all continued health and prosperity.
The Importance of Endpoint Security for Remote Work
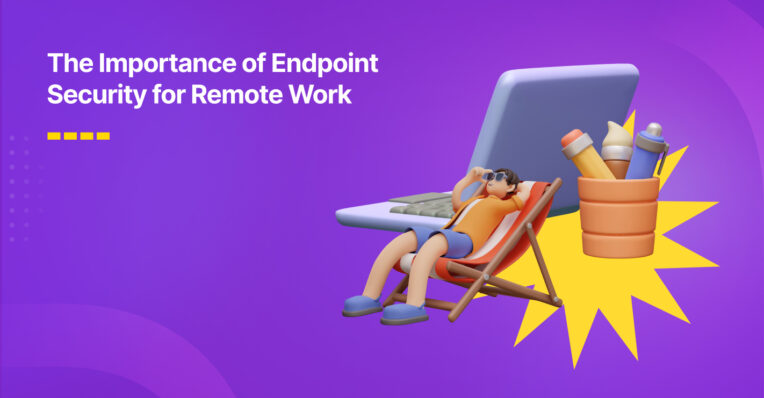
Remote work has revolutionised how we live and operate, offering unmatched flexibility and convenience. But with this shift comes a rising tide of cyber threats targeting the devices we rely on daily. Whether it’s your laptop at home, your smartphone at a coffee shop, or a tablet on the go, these endpoints are gateways to sensitive organisational data—and prime targets for hackers.
Protecting your remote work environment isn’t just about securing data; it’s about safeguarding trust, business continuity, and peace of mind.
This article explains why endpoint security is critical for remote work, highlighting key risks, real-world examples, and actionable best practices to help you stay protected.
Understanding Endpoint Security in the Remote Work Era
What Is Endpoint Security, and Why Does It Matter?
Endpoint security is like locking the doors and windows of your organisation’s digital house. It refers to the strategies, tools, and policies designed to protect devices connected to your network—whether it’s a corporate laptop, a personal smartphone, or a remote worker’s tablet.
These devices act as portals to sensitive information. Without proper security, a single compromised endpoint could expose your entire IT system to cyberattacks. In today’s blended work environment, where personal and professional devices often overlap, endpoint security has become essential for organisational survival.
How Remote Work Has Changed the Game
The rise of remote and hybrid work has reshaped endpoint security in profound ways. Key trends include:
- BYOD (Bring Your Own Device): Employees increasingly use personal devices for work, complicating device management and data protection.
- Unsecured Networks: Many remote workers access data over unprotected Wi-Fi networks, leaving sensitive information vulnerable to interception.
- Cloud-First Workflows: Heavy reliance on cloud applications creates additional risks as endpoints interact with external networks.
These challenges demand adaptable and robust endpoint security measures to manage diverse devices, behaviours, and workflows.
The Threats Lurking in Remote Work
Cyber Risks That Remote Workers Face
Cybercriminals are quick to exploit vulnerabilities in remote work setups. Common threats include:
- Phishing Attacks: Fraudulent emails or messages designed to steal credentials.
- Ransomware: Malicious software that encrypts files and demands payment for their release.
- Unsecured Devices: Devices lacking encryption or up-to-date security protocols, creating easy targets for hackers.
Real-World Lessons from Cybersecurity Breaches
1. National Data Centre Ransomware Attack (2024):
In June 2024, Indonesia’s National Data Centre suffered a major ransomware attack, disrupting services across more than 230 government agencies, including immigration and airport operations. The Brain Cipher group demanded an $8 million ransom but later released the decryption key without payment. This attack exposed vulnerabilities in government systems and emphasised the need for robust cybersecurity measures. Read more.
2. India’s Energy Sector Breach (2020–2021):
A series of cyberattacks targeted India’s power sector, including the National Thermal Power Corporation (NTPC) and a major power outage in Mumbai. The Chinese-backed hacker group, RedEcho, used advanced malware called ShadowPad to infiltrate systems via legitimate software updates. The attack caused significant disruption and data theft, highlighting the urgent need for robust endpoint security to protect critical infrastructure. Read more.
3. Canada’s Laptop Data Breach (2018):
A stolen laptop containing unencrypted data exposed the personal information of over 33,000 Canadians. The breach included sensitive details like social insurance numbers and birth dates, exposing weak compliance measures and poor encryption protocols. This incident serves as a reminder of how a single unprotected endpoint can lead to large-scale breaches. Read more.
These cases illustrate how endpoint vulnerabilities can lead to serious repercussions across industries.
Best Practices for Securing Endpoints in a Remote Setting
1. Educate Employees
Most cyberattacks begin with human error. Whether it’s clicking on a phishing link or using a weak password, employees can inadvertently open the door to threats. Regular training on recognising phishing attempts, securing personal devices, and following cybersecurity best practices can empower your workforce to be the first line of defence.
2. Strengthen IT Infrastructure with Endpoint Security
Effective endpoint security solutions should integrate seamlessly with your existing IT environment. Look for features like centralised management, scalability for organisational growth, and compatibility with Security Information and Event Management (SIEM) systems for real-time threat analysis.
3. Require Virtual Private Networks (VPNs)
VPNs create encrypted tunnels for data transmission, protecting sensitive information from prying eyes—especially when employees use unsecured public Wi-Fi. Mandating VPN use for remote workers is a simple yet effective way to reduce the risk of data breaches.
4. Conduct Regular Audits and Compliance Checks
Periodic audits uncover potential vulnerabilities and ensure compliance with data protection regulations like GDPR or HIPAA. Proactive assessments help identify weaknesses in endpoint security strategies and demonstrate your organisation’s commitment to protecting data.
5. Implement Multi-Factor Authentication (MFA)
Passwords alone aren’t enough to keep attackers out. Multi-Factor Authentication requires users to verify their identity with an additional layer, like a fingerprint, a code sent to their phone, or facial recognition. Even if a password is compromised, MFA can prevent unauthorised access, making it a cornerstone of endpoint security.
Conclusion: Keep Your Remote Work Environment Safe
Remote work has brought unparalleled flexibility—but also new security challenges. Protecting endpoints is no longer optional; it’s the backbone of a secure, productive remote work environment. By educating your team, strengthening IT Infrastructure with Endpoint Security, implementing tools like VPN and MFA, and staying proactive with audits, you can reduce risks and maintain trust in your organisation.
How Mitrais Powers Integrated Research's Success
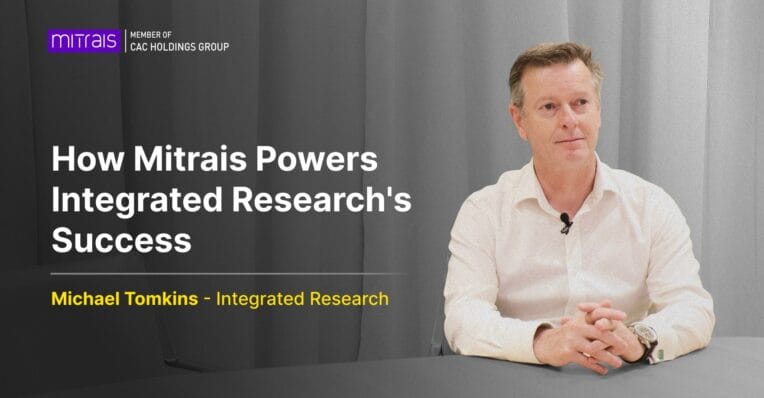
Imagine a partnership built on trust, innovation, and a shared commitment to excellence. Mitrais has been more than just a software development partner to Integrated Research (ASX:IRI), a leader in critical system communication, payment, and infrastructure monitoring.
It wasn’t just about writing code. Integrated Research significantly increased their development velocity while maintaining top-notch quality by partnering with Mitrais, as we focus on measurable outcomes and constant improvement. Plus, providing the right talent, perfectly matched to their needs, has been a key part of our long-term partnership.
Our General Manager, Rob Mills, and Michael Tomkins, Chief Technology Officer of Integrated Research highlight that our partnership is about building a strong, collaborative relationship.
Want to see how Mitrais ticks all the boxes for Integrated Research’s software development needs? Click here to watch the video now!
Transforming Employee Engagement with AI: Implementing an LLM Chatbot in Mitrais
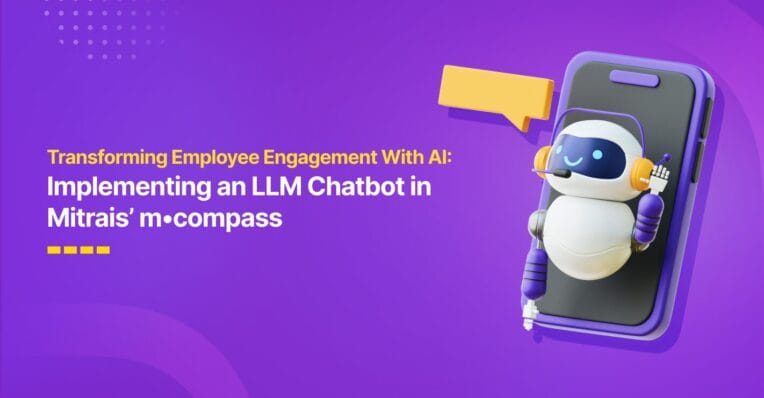
Abstract
In this white paper, we explore the implementation of a Large Language Model (LLM)-powered chatbot within the Competency System of our organisation. The chatbot utilises Retriever-Augmented Generation (RAG) embeddings to answer employee enquiries related to company policies, competencies, and other system-related queries. Additionally, function calling is integrated to dynamically fetch personalised employee details. This document highlights the technical aspects of the system, its operational benefits, challenges encountered, and the future potential of AI-driven HR support systems.
Introduction
With the rapid advancement of artificial intelligence, organisations are increasingly leveraging AI to streamline internal processes and improve employee experiences. A notable innovation in this space is the deployment of LLM-powered chatbots, which provide on-demand support and information to employees. In our organisation, we have integrated an LLM chatbot into the Competency System, allowing employees to access relevant information about their competencies, policies, and more, through natural language conversations.
This white paper outlines the technical implementation of this system, including the use of RAG embeddings for answering policy-related queries and function calling to retrieve employee-specific data, as well as the resulting impact on operational efficiency and employee satisfaction.
The Competency System and Its Challenges
The Competency System in our organisation serves as a central hub for employee development, containing critical information regarding job roles, required competencies, performance evaluations, and more. Traditionally, accessing this information required navigating through various manuals, policy documents, or interacting with HR representatives.
However, as the workforce grew and the volume of questions regarding the system increased, there arose a need for an efficient, scalable way to provide employees with answers to their enquiries. The integration of an LLM chatbot was seen as a strategic move to address this challenge by offering real-time, accurate responses based on our competency policies.
Solution Overview: Implementing the LLM Chatbot
LLM Chatbot Architecture
The chatbot is built upon a state-of-the-art LLM, capable of processing natural language queries and delivering coherent, contextually accurate responses. The system leverages the following components:
- Retriever-Augmented Generation (RAG) Embeddings: The LLM is enhanced with RAG embeddings, which help the model retrieve relevant information from a large corpus of policy documents and knowledge bases. This allows the system to answer employee questions about competencies, policies, and organisational procedures with high accuracy.
- Function Calling: To personalise responses and provide specific employee information (e.g., performance evaluations, training completion), the system calls on internal databases to fetch relevant data dynamically. This integration ensures that responses are not only contextually relevant but also tailored to the individual employee’s profile.
Workflow and Integration
The chatbot operates in real-time, processing employee queries and retrieving information from both static (policy documents) and dynamic (employee data) sources.
The process flow is detailed below:
Figure 1 M-Compass chatbot workflow
- The employee submits a question via the chatbot interface.
- The LLM, enhanced with RAG embeddings, parses the query and retrieves relevant information from internal policies and knowledge bases.
- If the query requires personalised data, function calls are made to fetch specific employee details from the internal system.
- The chatbot generates a coherent and personalised response, which is displayed to the employee.
Technical Details and Considerations
Challenges in Policy Retrieval
One of the primary challenges we faced was ensuring that the LLM could accurately retrieve and present information from a vast array of policy documents. This was addressed by using RAG embeddings, which combine traditional retrieval-based methods with generative language models to enhance the quality and relevance of the responses. The process begin with preprocessing the policy documents: they were segmented into manageable chunks (e.g., paragraphs or sections), tokenized, and converted into dense vector representations using a transformer-based embedding model. These embeddings were then indexed in a vector database optimized for fast similarity searches.
During query processing, the system first encodes the user’s question into a vector and retrieves the top-k most relevant document chunks based on cosine similarity or another distance metric. The LLM then uses this retrieved context to generate a coherent and contextually appropriate response, rather than relying solely on its pre-trained knowledge.
Integration with Employee Data Systems
Another significant challenge was ensuring seamless integration with internal systems to pull personalised employee data. Function calling was implemented to ensure secure and efficient retrieval of sensitive information while maintaining privacy and compliance with data protection regulations. The process likely involved defining a set of API endpoints each tied to a specific function, such as getEmployeeDetails(employeeID) or fetchCompetency(employeeID) that the LLM could invoke dynamically based on the query’s intent.
To implement this, the team uses LLM tool features to recognise when a query requires external data (e.g., “What’s my detail information?”) and map it to the appropriate function call. Data privacy was maintained by limiting data retrieval to the minimum necessary fields.
Ensuring Accuracy and Relevance
Given the complexity and variety of queries employees could ask, we focused on fine-tuning the LLM to handle diverse topics and provide precise answers. Continuous monitoring and adjustments were made to improve the accuracy of the responses, especially for more complex or ambiguous questions. We ensure relevance by incorporating user context wherever possible leveraging metadata like the employee’s role, or department to tailor responses. We also converted the policy document into Q&A format which could increase the accuracy of the responses.
Benefits and Impact
Enhanced Efficiency
The implementation of the LLM chatbot has drastically reduced the time HR staff spends answering repetitive or routine questions. Employees now have 24/7 access to the information they need, improving overall efficiency.
Improved Employee Satisfaction
The ability to quickly retrieve answers to competency-related questions has contributed to improved employee satisfaction and engagement. With shorter wait times and easier access to important information, employees are better supported in managing their professional development.
Scalability
The LLM chatbot has proven to be highly scalable, handling hundreds of employee enquiries without additional strain on HR resources. As the company grows, the system can easily accommodate more users and incorporate additional functionality.
Future Directions
As AI technology continues to evolve, there are several potential avenues for further improving the system:
- Enhanced Personalisation: Leveraging deeper integrations with employee performance data and development goals could allow the system to offer more tailored career advice and insights.
- Continuous Learning: The system could be further enhanced with continuous learning capabilities, allowing it to adapt to changes in policies and employee needs over time.
Conclusion
The implementation of an LLM chatbot in our Competency System represents a significant step forward in leveraging Artificial Intelligence to improve employee engagement, streamline HR processes, and increase operational efficiency. By combining RAG embeddings and function calling, we have created a system that not only provides accurate policy-based answers but also personalises responses based on individual employee data. As the system evolves, we are confident it will continue to transform the way our employees interact with the Competency System, driving greater satisfaction and fostering a more agile workforce.
Abstract
In this white paper, we explore the implementation of a Large Language Model (LLM)-powered chatbot within the Competency System of our organisation. The chatbot utilises Retriever-Augmented Generation (RAG) embeddings to answer employee enquiries related to company policies, competencies, and other system-related queries. Additionally, function calling is integrated to dynamically fetch personalised employee details. This document highlights the technical aspects of the system, its operational benefits, challenges encountered, and the future potential of AI-driven HR support systems.
Introduction
With the rapid advancement of artificial intelligence, organisations are increasingly leveraging AI to streamline internal processes and improve employee experiences. A notable innovation in this space is the deployment of LLM-powered chatbots, which provide on-demand support and information to employees. In our organisation, we have integrated an LLM chatbot into the Competency System, allowing employees to access relevant information about their competencies, policies, and more, through natural language conversations.
This white paper outlines the technical implementation of this system, including the use of RAG embeddings for answering policy-related queries and function calling to retrieve employee-specific data, as well as the resulting impact on operational efficiency and employee satisfaction.
The Competency System and Its Challenges
The Competency System in our organisation serves as a central hub for employee development, containing critical information regarding job roles, required competencies, performance evaluations, and more. Traditionally, accessing this information required navigating through various manuals, policy documents, or interacting with HR representatives.
However, as the workforce grew and the volume of questions regarding the system increased, there arose a need for an efficient, scalable way to provide employees with answers to their enquiries. The integration of an LLM chatbot was seen as a strategic move to address this challenge by offering real-time, accurate responses based on our competency policies.
Solution Overview: Implementing the LLM Chatbot
LLM Chatbot Architecture
The chatbot is built upon a state-of-the-art LLM, capable of processing natural language queries and delivering coherent, contextually accurate responses. The system leverages the following components:
- Retriever-Augmented Generation (RAG) Embeddings: The LLM is enhanced with RAG embeddings, which help the model retrieve relevant information from a large corpus of policy documents and knowledge bases. This allows the system to answer employee questions about competencies, policies, and organisational procedures with high accuracy.
- Function Calling: To personalise responses and provide specific employee information (e.g., performance evaluations, training completion), the system calls on internal databases to fetch relevant data dynamically. This integration ensures that responses are not only contextually relevant but also tailored to the individual employee’s profile.
Workflow and Integration
The chatbot operates in real-time, processing employee queries and retrieving information from both static (policy documents) and dynamic (employee data) sources.
The process flow is detailed below:
Figure 1 M-Compass chatbot workflow
- The employee submits a question via the chatbot interface.
- The LLM, enhanced with RAG embeddings, parses the query and retrieves relevant information from internal policies and knowledge bases.
- If the query requires personalised data, function calls are made to fetch specific employee details from the internal system.
- The chatbot generates a coherent and personalised response, which is displayed to the employee.
Technical Details and Considerations
Challenges in Policy Retrieval
One of the primary challenges we faced was ensuring that the LLM could accurately retrieve and present information from a vast array of policy documents. This was addressed by using RAG embeddings, which combine traditional retrieval-based methods with generative language models to enhance the quality and relevance of the responses. The process begin with preprocessing the policy documents: they were segmented into manageable chunks (e.g., paragraphs or sections), tokenized, and converted into dense vector representations using a transformer-based embedding model. These embeddings were then indexed in a vector database optimized for fast similarity searches.
During query processing, the system first encodes the user’s question into a vector and retrieves the top-k most relevant document chunks based on cosine similarity or another distance metric. The LLM then uses this retrieved context to generate a coherent and contextually appropriate response, rather than relying solely on its pre-trained knowledge.
Integration with Employee Data Systems
Another significant challenge was ensuring seamless integration with internal systems to pull personalised employee data. Function calling was implemented to ensure secure and efficient retrieval of sensitive information while maintaining privacy and compliance with data protection regulations. The process likely involved defining a set of API endpoints each tied to a specific function, such as getEmployeeDetails(employeeID) or fetchCompetency(employeeID) that the LLM could invoke dynamically based on the query’s intent.
To implement this, the team uses LLM tool features to recognise when a query requires external data (e.g., “What’s my detail information?”) and map it to the appropriate function call. Data privacy was maintained by limiting data retrieval to the minimum necessary fields.
Ensuring Accuracy and Relevance
Given the complexity and variety of queries employees could ask, we focused on fine-tuning the LLM to handle diverse topics and provide precise answers. Continuous monitoring and adjustments were made to improve the accuracy of the responses, especially for more complex or ambiguous questions. We ensure relevance by incorporating user context wherever possible leveraging metadata like the employee’s role, or department to tailor responses. We also converted the policy document into Q&A format which could increase the accuracy of the responses.
Benefits and Impact
Enhanced Efficiency
The implementation of the LLM chatbot has drastically reduced the time HR staff spends answering repetitive or routine questions. Employees now have 24/7 access to the information they need, improving overall efficiency.
Improved Employee Satisfaction
The ability to quickly retrieve answers to competency-related questions has contributed to improved employee satisfaction and engagement. With shorter wait times and easier access to important information, employees are better supported in managing their professional development.
Scalability
The LLM chatbot has proven to be highly scalable, handling hundreds of employee enquiries without additional strain on HR resources. As the company grows, the system can easily accommodate more users and incorporate additional functionality.
Future Directions
As AI technology continues to evolve, there are several potential avenues for further improving the system:
- Enhanced Personalisation: Leveraging deeper integrations with employee performance data and development goals could allow the system to offer more tailored career advice and insights.
- Continuous Learning: The system could be further enhanced with continuous learning capabilities, allowing it to adapt to changes in policies and employee needs over time.
Conclusion
The implementation of an LLM chatbot in our Competency System represents a significant step forward in leveraging Artificial Intelligence to improve employee engagement, streamline HR processes, and increase operational efficiency. By combining RAG embeddings and function calling, we have created a system that not only provides accurate policy-based answers but also personalises responses based on individual employee data. As the system evolves, we are confident it will continue to transform the way our employees interact with the Competency System, driving greater satisfaction and fostering a more agile workforce.
I Putu Arya Kusuma Wijaya: Navigating the Cyber Seas with Mitrais
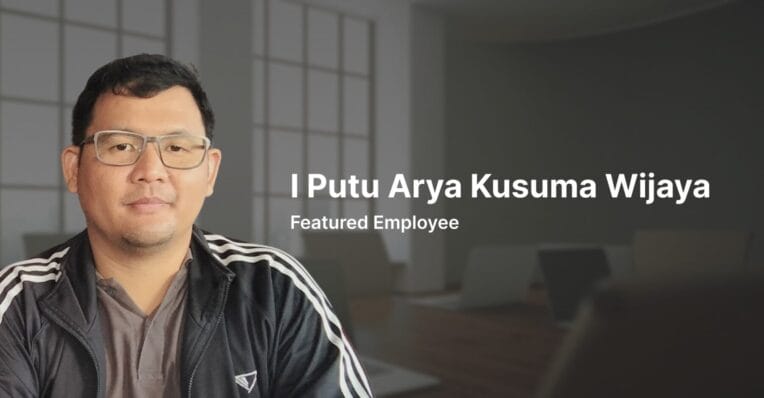
This issue we take the time to introduce I Putu Arya Kusuma Wijaya, known fondly as Arya. Hailing from Denpasar, Arya’s journey into the world of technology and cybersecurity is an inspiring and impressive one.
Arya’s academic journey began with a bachelor’s degree in electrical engineering from Udayana University. Eager to carve out a niche in the IT world, he has pursued an array of added certifications, including Security+, Azure, AWS, CISA, and CISSP. These credentials not only broadened his expertise but also prepared him for the dynamic challenges of the cybersecurity realm.
Arya’s professional career landed him at Mitrais, the leading IT company in Bali. Initially joining as part of our Information and Communications Technology (Infocomm) team, Arya’s dedication and proven skills soon earned him promotions, and he now serves as the Compliance and Operations Lead. In this role, he lends his considerable abilities to IT operations, desktop support, network monitoring, and ISO 27001 compliance.
His passion for cybersecurity was ignited in 2017 when he embarked on his first training with CompTIA (one of the world’s most trusted certifying bodies) in Security+. This marked the beginning of a journey filled with learning and professional development. As an ISO 27001 implementer at Mitrais, Arya continues to refine his skills and stay abreast of industry advancements through added certifications.
Reflecting on his ambitions, Arya says that it is remarkable how his dreams steadily evolved from simply creating games to aspiring for leadership. His current goal is to become an exemplary leader, guiding his team towards success and continuous growth.
One of the aspects Arya appreciates most about Mitrais is the company’s culture. He finds the team’s humility and friendliness refreshing, and he enjoys the continuous learning environment that Mitrais fosters. This supportive atmosphere has been instrumental in his professional growth and achievements.
Implementing ISO certification for the first time posed some significant challenges for Arya’s team, but with the unwavering support of Mitrais management and the proactive approach of his colleagues, they successfully navigated the rigorous certification process. This collaborative effort ensured that the company met (and still meets) all necessary standards and achieved that valuable certification, with all the benefits that our clients gain from that.
Arya’s journey is a testament to the power of ambition, continuous learning, and teamwork. Looking forward, he is still committed to his goal of becoming a successful and productive leader within Mitrais. His story no doubt serves as an inspiration to many, highlighting how dedication and a supportive environment can pave the way for remarkable achievements in the IT and cybersecurity fields.
But Arya’s story is not just about individual success; it’s about the collective spirit of Mitrais that nurtures talent and drives growth. As Arya continues to excel and inspire, we can only anticipate more extraordinary milestones in his journey, and Mitrais and our clients benefit from that.